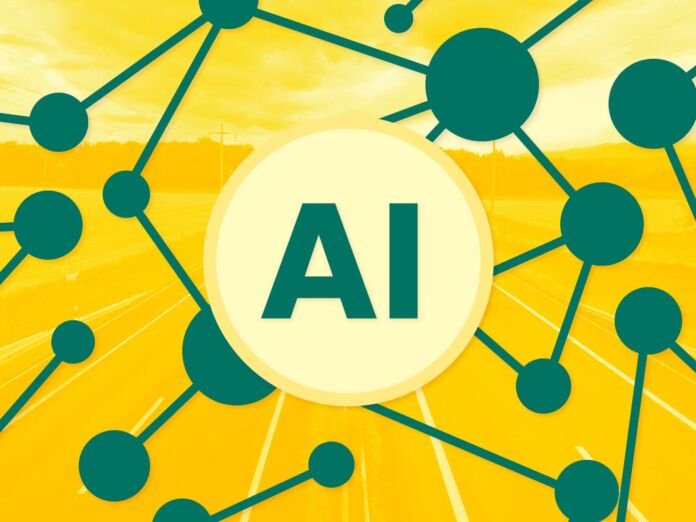
It’s a timeless manufacturing goal: producing more, higher-quality products at minimum cost. The Smart Manufacturing revolution is already enabling manufacturers to reach this goal more successfully than ever. And one of the core technologies driving this wave of innovation is Artificial Intelligence. Data has become a highly valuable resource, and it’s cheaper than ever to capture and store. Today, more manufacturers than ever before are leveraging that data to significantly improve their bottom line thanks to Artificial Intelligence (AI) – and particularly Machine Learning (ML).
For many, this means greatly improving production efficiency and capacity by eliminating the primary causes of production losses and other associated costs. Of course, getting tangible business value out of AI is often easier said than done. It is a complex technology, with many different applications. How can manufacturers see through the “hype” and empty promises to invest in Industrial AI that will truly give them a competitive edge?
“Today, more manufacturers than ever before are leveraging that data to significantly improve their bottom line thanks to Artificial Intelligence (AI) – and particularly Machine Learning (ML).“
-Augury
The Key to Success With AI and ML
It’s impossible to miss the rapid rise of AI technology – both in general and specifically in the context of manufacturing. As a result, the expectations of AI are often wildly off-base, varying from an all-encompassing solution to fix your business problems or a deep skepticism any time AI is even uttered.
Find the Right Use Case
But, as with any technology, the truth is really somewhere in between. AI can be extremely effective – in the right context. Understanding those contexts, and the kinds of AI technology that apply to them, is the key to setting realistic business goals for AI adoption.
Artificial Intelligence is not a silver bullet. No solution will solve all, or most, of your problems. As a rule of thumb, AI works best when it is applied to solving a specific problem, or a very closely-related set of problems.
General AI is something to be wary of: if a vendor claims to do everything, then they probably do nothing particularly well. This brings us back to the topic of AI in manufacturing. There are numerous potential applications for AI and Machine Learning in manufacturing, and each use case requires a unique type of Artificial Intelligence.
The guide below presents a simple, effective formula for selecting the right Industrial AI solution to address specific manufacturing challenges and goals.
The focus remains specifically on Machine Learning AI since that is where the most exciting and impactful innovations are occurring. The formula can be encapsulated in a simple diagram and methodology called, “The Industrial AI Quadrant.”
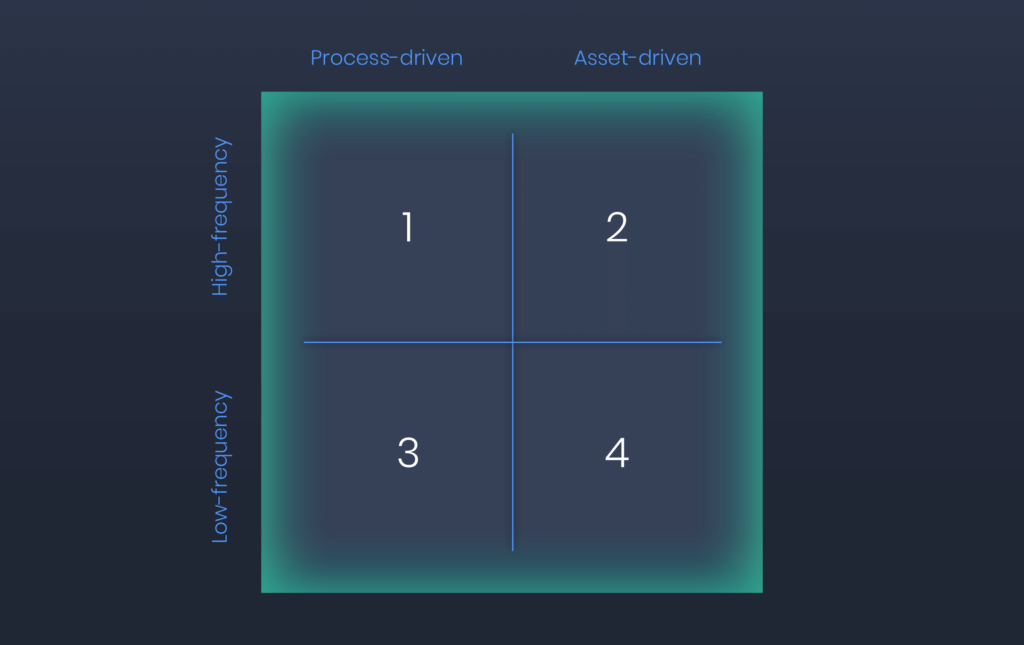
Next-Generation Optimization With ML
The two major use cases of Machine Learning in manufacturing are Predictive Quality & Yield and Predictive Maintenance.
#1: Only Do Maintenance When Necessary
Predictive Maintenance is the more commonly known of the two, given the significant costs maintenance issues and associated problems can incur, which is why it is now a fairly common goal amongst manufacturers.
Instead of performing maintenance according to a predetermined schedule, or using SCADA systems set up with human-coded thresholds, alert rules, and configurations, predictive maintenance uses algorithms to predict the next failure of a component/machine/system. Personnel can then be alerted to perform focused maintenance procedures to prevent failure, but not too early so as to waste unnecessary downtime.
By contrast, traditional manual and semi-manual approaches don’t take into account the more complex dynamic behavioral patterns of the machinery, or the contextual data relating to the manufacturing process at large. For example, a sensor on a production machine may pick up a sudden temperature rise. A static rule-based system would not take into account the fact that the machine is undergoing sterilization, and would proceed to trigger a false-positive alert.
The advantages are numerous and can significantly reduce costs while eliminating the need for planned downtime in many cases.
By preempting a failure with a machine learning algorithm, systems can continue to function without unnecessary interruptions. When maintenance is needed, it’s very focused – technicians are informed of the components that need inspection, repair, and replacement; which tools to use, and which methods to follow.
Predictive maintenance also leads to a longer Remaining Useful Life (RUL) of machinery and equipment since secondary damage is prevented while smaller labor forces are needed to perform maintenance procedures.
#2: Find the Hidden Causes of Losses
Predictive Quality and Yield – sometimes referred to as just Predictive Quality – is a more advanced use case of Industrial AI that reveals the hidden causes of many of the perennial process-based production losses manufacturers face daily. Examples include quality, yield, waste, throughput, energy efficiency, emissions, and more – essentially any loss caused by process inefficiencies.
Predictive Quality and Yield automatically identify the root causes of process-driven production losses using continuous, multivariate analysis, powered by Machine Learning algorithms that are uniquely trained to intimately understand each production process.
Automated recommendations and alerts can then be generated to inform production teams and process engineers of an imminent problem, and seamlessly share important knowledge on how to prevent losses before it occurs.
Reducing these types of losses has always been a struggle for manufacturers of all stripes. But in today’s marketplace, this mission is critical. On the one hand, consumers’ expectations are at an all-time high; global consumer habits are gradually “westernizing,” even as the population boom continues.
According to numerous surveys, the global population will grow by 25 percent by 2050, equating to some 200,000 additional mouths to feed every day. On the other hand, consumers have never had so many alternatives available to them, in almost every product imaginable.
Recent surveys indicate that this wealth of options means consumers are increasingly likely to permanently ditch even their favorite brands if, for example, a product isn’t available on the shelf.
Against such a backdrop, manufacturers can no longer afford to take process inefficiencies, and their associated losses, in their stride. Every loss in terms of waste, yield, quality, or throughput chips away at their bottom line and hands another inch to the competition.
The challenge for many manufacturers is that they eventually hit a glass ceiling in terms of process optimization. Some inefficiencies don’t have any obvious cause, and process experts are left at a loss to explain them. That’s where Machine Learning – and particularly Automated Root Cause Analysis – can save the day.
Two Main Categories of Machine Learning
Machine Learning can be split into two main techniques: Supervised and Unsupervised.
#1: Supervised Machine Learning
In manufacturing use cases, supervised machine learning is the most commonly used technique since it leads to a predefined target: we have the input data; we have the output data; and we’re looking to map the function that connects the two variables.
Supervised ML demands a high level of involvement – data input, data training, defining, and choosing algorithms, data visualizations, and so on. The goal is to construct a mapping function with a level of accuracy that allows teams to predict outputs when new input data is entered into the system.
Initially, the algorithm is fed from a training dataset, and by working through iterations, continues to improve its performance as it aims to reach the defined output. The learning process is completed when the algorithm reaches an acceptable level of accuracy.
In manufacturing, there are two most common Supervised Learning approaches: Regression & Classification. These two approaches share the same goal: to map a relationship between the input data (from the manufacturing process) and the output data (known possible results such as quality or waste losses, part failure, overheating, etc).
Regression
Regression is used when data exists within a range (eg. temperature, weight), which is often the case when dealing with data collected from sensors. In manufacturing, a regression can be used to calculate an estimate for the RUL of an asset. This is a prediction of how many days or cycles we have before the next component/machine/system failure.
For regression, the most commonly used machine learning algorithm is Linear Regression, being fairly quick and simple to implement, with an output that is easy to interpret. An example of linear regression would be a system that predicts temperature since temperature is a continuous value with an estimate that would be simple to train.
Classification
When data exists in well-defined categories, classification can be used. An example of classification that most are familiar with is the email filter algorithm that decides whether an email should be sent to the spam folder, or not.
Classification is limited to a boolean value response but can be very useful since only a small amount of data is needed to achieve a high level of accuracy. In ML, common classification algorithms include naive Bayes, logistic regression, support vector machines, and Artificial Neural Networks.
#2: Unsupervised ML
Supervised machine learning starts by working from an expected outcome and trains the algorithm accordingly. Unsupervised learning is suitable for cases where the outcome is not yet known.
Clustering
In some cases, not only will the outcome be unknown, but information describing the data will also be lacking (data labels). By creating clusters of input data points that share certain attributes, a Machine Learning algorithm can discover underlying patterns. Clustering can also be used to reduce noise (irrelevant parameters within the data) when dealing with extremely large numbers of variables.
Artificial Neural Networks
In the manufacturing sector, Artificial Neural Networks are proving to be an extremely effective unsupervised learning tool for a variety of applications including production process simulation and Predictive Quality Analytics.
The basic structure of the Artificial Neural Network is loosely based on how the human brain processes information using its network of around 100 billion neurons, allowing for extremely complex and versatile problem-solving.
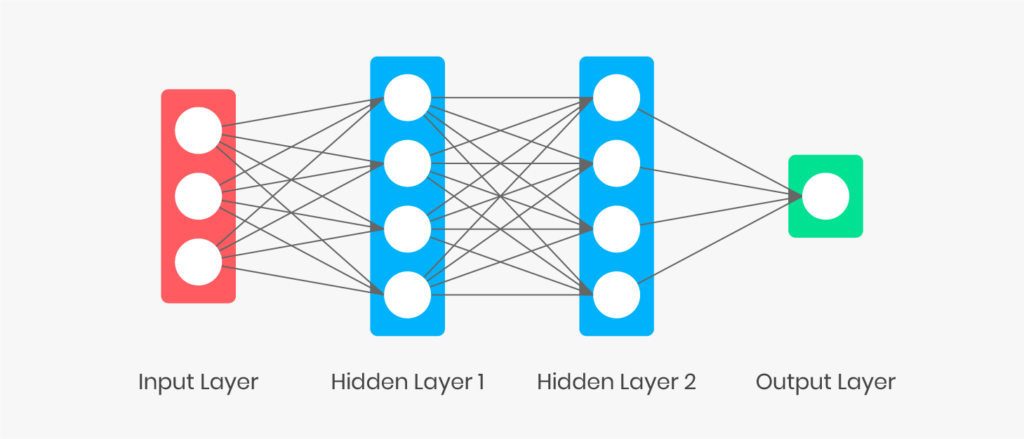
This ability to process a large number of parameters through multiple layers makes Artificial Neural Networks very suitable for the variable-rich and constantly changing processes common to manufacturing. Moreover, once properly trained, an Artificial Neural Network can demonstrate a high level of accuracy when creating predictions regarding the mechanical properties of processed products, enabling cuts in the cost of raw materials.
Data Preparation: Garbage In, Garbage Out
Machine Learning is all about data, so understanding some key elements about the quality and type of data needed is extremely important in ensuring accurate results. Predictive Quality and Yield, for example, focuses on process inefficiencies. Therefore, it makes sense to start by collecting historical data about the performance of the line or lines in question, as well as the losses incurred over time, to form predictions about future potential losses.
To get the most accurate picture possible, that data should be gathered from as many sources as possible, since manufacturing processes – especially more complex ones – are affected by a very wide range of factors that are often interdependent. This can include everything from process data, quality data, raw materials, and even external factors like weather and temperature.
Next, and just as importantly, one needs to decide what question the Machine Learning model should answer – and whether it is possible to answer this question using the data that’s available.
The Groundbreaking Benefits of AI and ML for Manufacturing
The introduction of AI and ML to industry represents a sea change with many benefits that can result in advantages well beyond efficiency improvements, opening doors to new business opportunities.
Some of the direct benefits of Machine Learning in manufacturing include:
- Reducing common, painful process-driven losses such as yield, waste, quality, and throughput
- Increased capacity by optimizing the production process.
- Enabling growth and expansion of product lines at scale due to a more optimized process.
- Cost reduction through Predictive Maintenance. PdM leads to less maintenance activity, which means lower labor costs and reduced inventory and materials wastage.
- Predicting Remaining Useful Life (RUL). Knowing more about the behavior of machines and equipment leads to creating conditions that improve performance while maintaining machine health. Predicting RUL does away with “unpleasant surprises” that cause unplanned downtime.
- Improved supply chain management through efficient inventory management and a well-monitored and synchronized production flow.
- Improved Quality Control with actionable insights to constantly raise product quality.
- Improved Human-Robot collaboration improving employee safety conditions and boosting overall efficiency.
- Consumer-focused manufacturing – being able to respond quickly to changes in market demand.
To get the most out of an Industrial Artificial Intelligence/Machine Learning solution, manufacturers need to know which AI solution is best suited for their own unique sets of challenges.
Tweet
Share
Share
- Artificial Intelligence
- Machine Learning
- Manufacturing
- Predictive Analytics
- Predictive Maintenance
- Artificial Intelligence
- Machine Learning
- Manufacturing
- Predictive Analytics
- Predictive Maintenance